At ProCogia, our unwavering commitment to advancing knowledge and solving complex challenges extends to the dynamic realms of bioinformatics and life sciences. Harnessing the power of cutting-edge technologies, we specialize in providing innovative solutions that propel scientific discovery and therapeutic advancements. From decoding intricate biological data to revolutionizing genomic analysis, ProCogia stands at the forefront of bioinformatics, utilizing data science expertise to unlock new insights and accelerate breakthroughs in life science research. Our mission is to empower researchers, clinicians, and biotech pioneers with transformative tools, making strides towards a healthier and more sustainable future. Emerging diseases like COVID-19 pose dual threats to public health and the economy. Understanding protein-protein interactions (PPIs) between viral and host proteins is crucial for antiviral therapies and studying pathogen replication. However, experimental techniques have limitations and machine learning models primarily focus on sequence-derived features, neglecting semantic information and necessitating effective encoding schemes. To address these challenges, we present DeProViR, a deep-learning framework for predicting virus-human interactions using amino acid sequences. DeProViR incorporates a Siamese-like neural network that combines convolutional and bidirectional LSTM networks to capture contextual information. Using GloVe embeddings, DeProViR seamlessly integrates semantic associations, enhancing PPI prediction. This innovative framework overcomes limitations in feature engineering and encoding scheme dependence. DeProViR provides an efficient solution for predicting host-virus interactions, facilitating therapy development, and advancing our understanding of diseases.
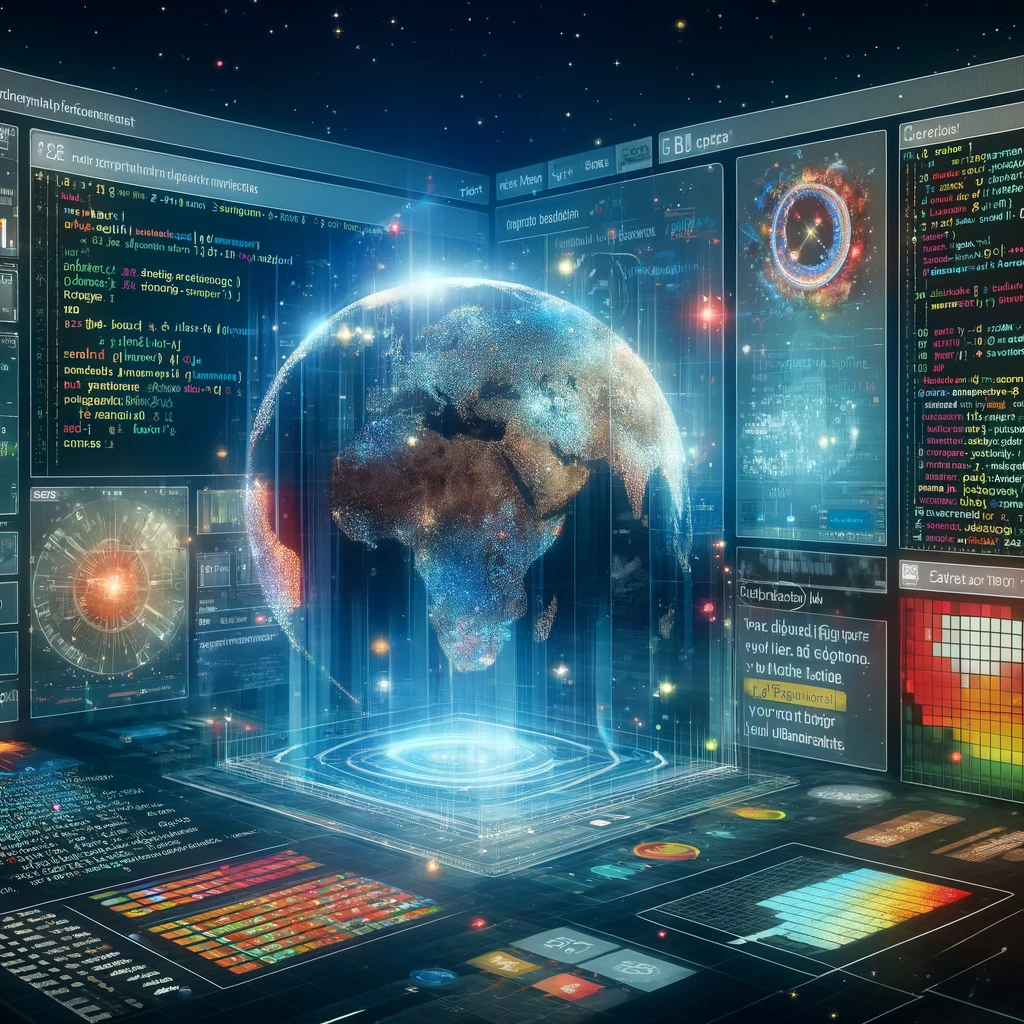
The Best IDE for Data Science Still Needs to be Built
Introduction Data science involves many programming languages and each comes with its preferred integrated development environment (IDE). I know this because I’ve been doing it